How to Solve Complex Tasks with a Generative AI-Enabled Hybrid Architecture
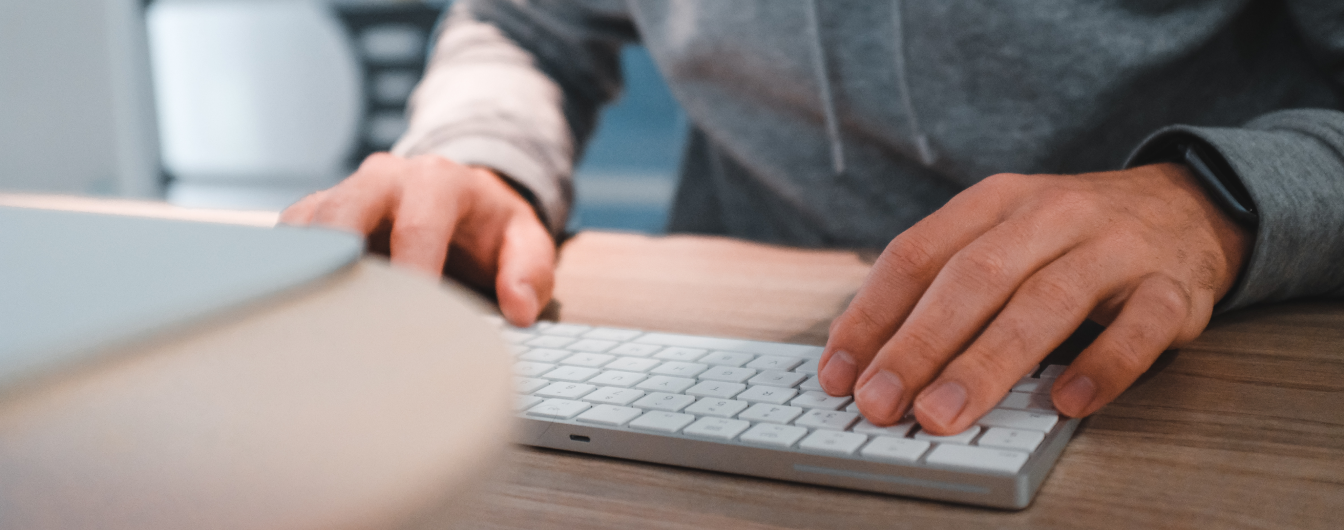
With the immense complexity of enterprises today, the amount of information available and variety of tools across the business are often siloed or even duplicated. Being able to find answers to complicated questions and solve complex tasks is often difficult, especially under time and resource constraints. This is where a systematic approach for AI-enabled decision making can help.
By now, you’ve probably heard of the generative AI product ChatGPT and, most likely, experimented with it. In the last few months, the wonders and dangers of this new tool have been discussed by governments and lawmakers, educators and students, tech and business crowds, teenagers and their grandparents. The latest advances in large language models (LLMs), like ChatGPT, have demonstrated the possibilities of what the future of generative AI could look like for businesses that are willing to embrace innovation. It is also clear that the rate of innovation in this space is not only greater than in previous years, but it is also more disruptive.
Clearly, LLMs have demonstrated their effectiveness in solving numerous natural language problems. However, it is important to acknowledge their limitations when it comes to handling complex, multi-step tasks, which can arise from the multi-nodal nature of these tasks or the associated costs involved. Solving multi-step tasks often requires a complex ecosystem of AI models and their enabling technologies and services to drive real incremental value for businesses. An anecdotal example in this paper — how to accurately count zebras across three photos – illustrates this complexity. Counting zebras is a straightforward task for us humans, but when it comes to AI, things become much more complex. Counting zebras then requires the use of image captioning, object detection, text classification, natural language processing and computer vision to accurately complete this task.
LLMs can be the orchestrators of that complexity and that’s where we’d like to focus — on a great example that can be found in the same paper we referenced earlier, which outlines how an existing wide range of models hosted on Hugging Face Hub can be employed, with the help and guidance of LLMs, to tackle complex tasks. This approach leverages the analytical and communication powers of LLMs and the wealth of pre-trained task-specific machine learning (ML) models to solve tasks across four stages — task planning, model selection, task execution and response generation.
To differentiate in the market today, businesses need to have the right ecosystem, enabling technologies and data assets in place to bring the power of AI to life. Below we’ll provide insight into what we believe are the most important components of that ecosystem and where you should begin your AI journey…
Spis treści
Laying the Groundwork
Before we discuss how LLMs can lead to real value in orchestrating your complex ecosystem and optimizing your decision-making processes, we must establish the key components required for a data framework to perform at its full potential. These include:
- A complete and up-to-date data catalogue and feature store, which are important components of any successful data processing as they serve as a centralized inventory of all available data assets. The inventory makes the data available and discoverable for correct use, ensuring that businesses can effectively manage and utilize their data assets.
- A model registry or centralized repository for managing and versioning ML models. The model registry keeps track of available models and maintains current information about them, including their domain, areas of applicability, data needs, performance and accuracy metrics, hyperparameters and limitations. Once again, this information makes models discoverable and available for a business’ ML flow.
- A decision engine, which is needed to automate decision-making processes. For instance, it can integrate responses from multiple AI models, query underlying data sources in near real time and make decisions based on this information, while also considering other relevant factors.
Using an LLM as a Data Governance Specialist
Maintaining an up-to-date and complete data catalogue, feature store and model repository can be a challenging and time-consuming task, especially in large enterprises. The volume of incoming information from multiple sources, along with data quality, data privacy and regulatory compliance, can add complexity to the process. Without proper management and maintenance, data catalogues and model repositories can become unreliable and outdated, leading to inaccurate or incomplete insights.
To help address these challenges, LLMs can act as a data governance specialist at your organization to perform the following tasks:
- Creating descriptions and metadata for datasets, their dependencies and features, as well as for models in the model repository. For datasets, LLMs can pull information from the dataset’s source, structure, dependencies and the features it contains. For models, LLMs can leverage the model’s areas of expertise, performance and accuracy metrics, datasets used in training and testing, and version control to generate descriptions.
- Assessing data quality and recency. LLMs can use natural language processing (NLP) algorithms to extract insights from unstructured data sources, such as customer feedback or social media posts.
- Controlling data and model redundancy. LLMs can identify and eliminate extra data and models in the catalog and repository, ensuring that the data and models are up-to-date and that resources are not wasted.
- Vectorizing tasks, datasets, features and model information. This enables efficient and accurate search capabilities based on natural language queries or other search criteria.
LLMs can also benefit decision engines in several ways. One of the key advantages is their ability to provide real-time, contextual information that can inform decisions and improve the accuracy and efficiency of the decision engine. With the ability to analyze unstructured data sources (such as social media, news articles and customer feedback) in real time, LLMs can identify emerging trends, sentiments and other factors that can impact decision making. This can be especially valuable in situations where speed and accuracy are critical, like financial trading, fraud detection or emergency response.
Leveraging Generative AI to Solve Real-Life Complex Business Tasks
Now that we have established the essential practices and procedures required to keep data, models and decision-making processes up-to-date, available and easily discoverable, we can examine the process of solving complex tasks and where LLMs can help. These four steps are the same as where we began with this blog, but with the right data foundation and ecosystem in place, can yield more effective results:
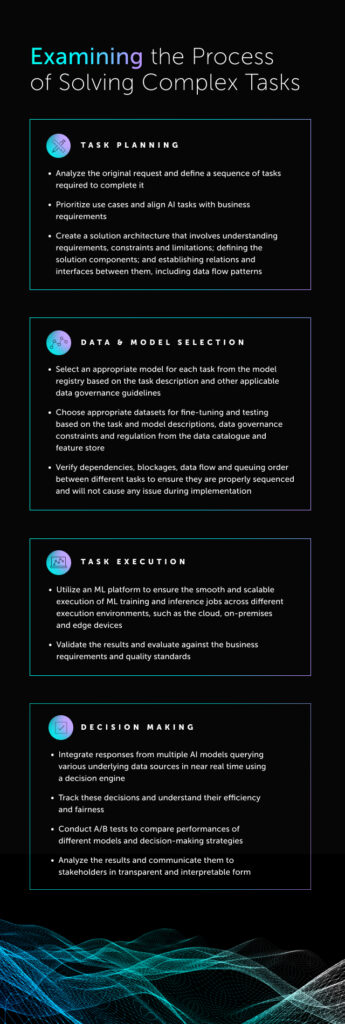
As we discussed previously, there is more to any solution than simply making a clever choice of data and models and coordinating and executing them effectively. Real-world solutions typically involve multiple services and environments and must adhere to numerous rules and constraints, such as privacy-preserving measures. To address these constraints, we suggest a hybrid (hub and spokes) architecture that leverages a single generalized model to coordinate tasks between specialized models, internal and external data sources and compute services. This architecture effectively integrates services and environments, while also addressing security and data confidentiality concerns.
In Conclusion
The recent advancements in LLMs have opened new opportunities for AI problem-solving at scale. With their improved and expanding capabilities, these models have revolutionized the field of AI, making it possible to tackle complex problems in new and innovative ways. To leverage the full potential of AI, you need to:
- First, ensure you have the right components required for a data framework to perform at its fullest potential.
- Use the data governance capabilities of LLMs to ensure proper maintenance and management.
- Implement a hybrid architecture that leverages a single generalized model to coordinate tasks and effective integrate services and environments.
With this technology ecosystem in place, along with a focus on ML governance and responsible AI initiatives, you can enable discovery and query using natural language. This will allow you to generate synthetic test data on demand and control data quality.
AI development is constantly evolving and the number of ML models at your disposal could quickly reach the hundreds or thousands. To differentiate your business in the market, you need to orchestrate multiple components, models and technologies to benefit from the power of AI. We are here to discuss the ways in which you can reach this goal faster — at a moment when time is of the utmost importance.
This article was originally published on epam.com/insights . The article’s main photo is from envato.com.
Podobne artykuły
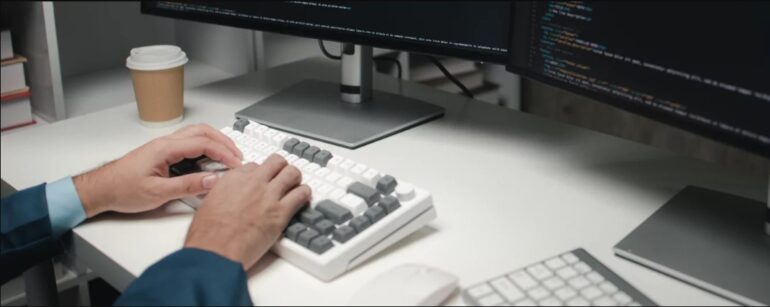
Transforming Quality Engineering with AI, ML and Big Data
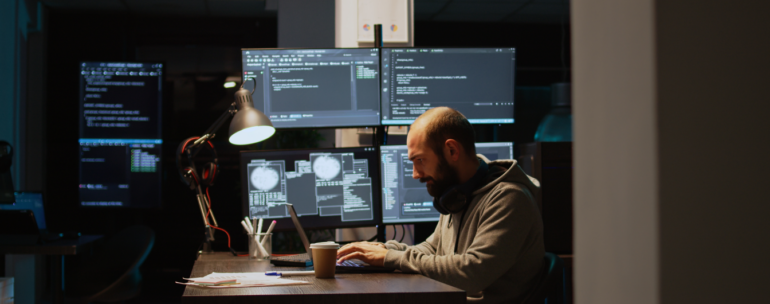
How to be a better software developer. Four things no one ever mentioned
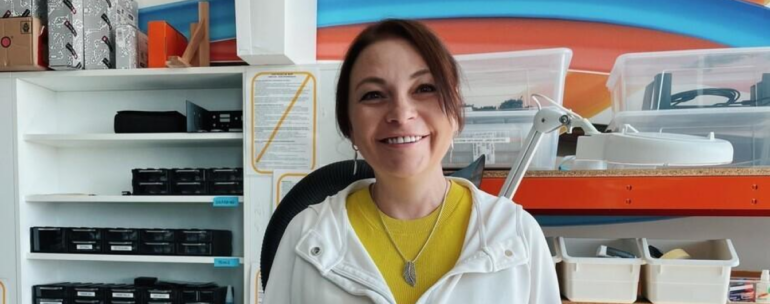
EPAM Team in the Final for NASAs MarsXR2 Challenge. Interview with Nataliia Savchenko
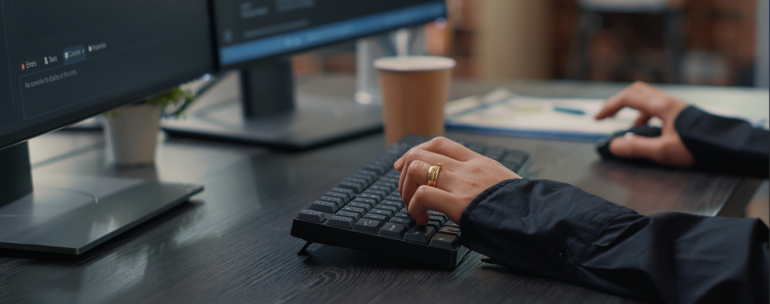